AI has been hailed as a silver bullet and a game-changing breakthrough in the modern economy. Like every new technology before it, AI captures the imagination as much as new businesses try to capture their share of the market. But the main question that many startups fail to answer is how to market, price, and sell their brand of AI technology? Instead look at integration into products as a way to monetize AI.
Background
AI has been hailed as a silver bullet and a game-changing breakthrough in the modern economy. Like every new technology before it, AI captures the imagination as much as new businesses try to capture their share of the market. While understanding what AI is important, this blog will show you that science is not where the money is. The money is in engineering solutions with that new science. Like if you could make base design work instant and augment human work. Or if we could use AI to make medical imaging and detection faster with less doctors. Let’s use this blog to define what intelligence, AI, and hardware opportunities are as a starting point within the context of entrepreneurship.
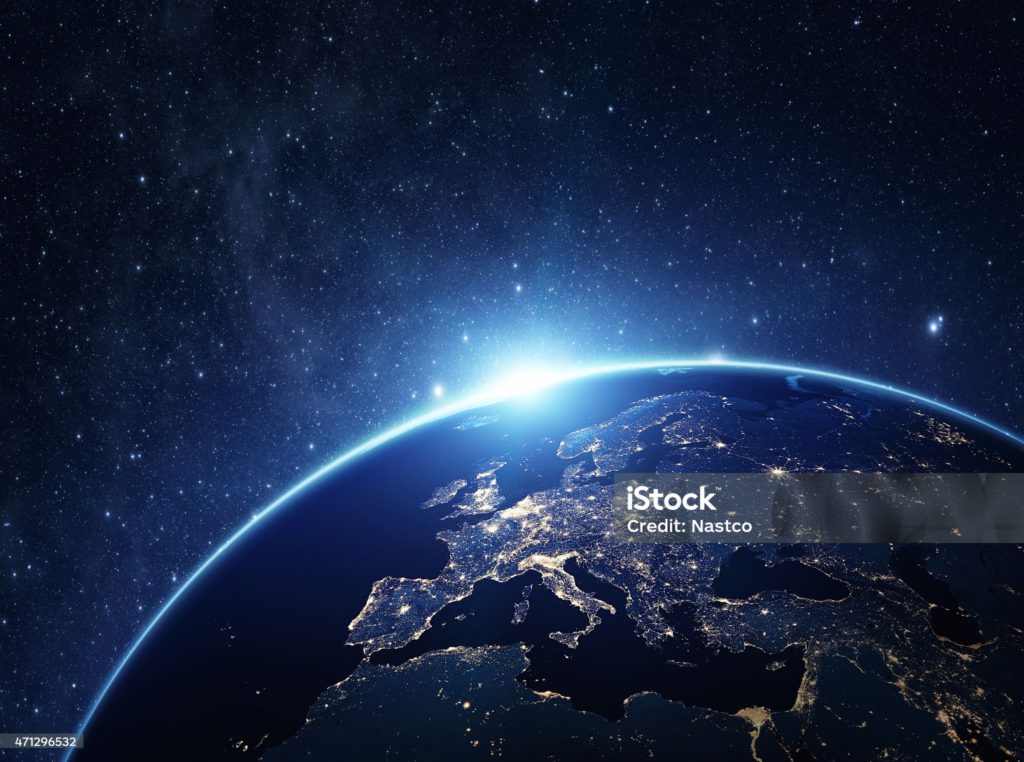
WHAT IS INTELLIGENCE?
Merriam-Webster defines intelligence as “the ability to learn or understand or to deal with new or trying situations”. This means the goal is to go beyond memorization or regurgitation to prove actual intelligence.
WHAT IS AI?
No matter the level of complication and sophistication, all AI is based off statistics: learning patterns and using those patterns to predict answers to new questions. The more advanced the patterns learned and statistics employed the more human the AI feels to the user. But, the complicated nature of the statistics can lead to unexpected results, only because humans have a tough time realizing patterns in large data. This makes it very difficult to design a predictable AI and capture corners in its design.
A computer can easily memorize and store knowledge and data, but that is not intelligence. Today’s AI goes beyond just a file system, but does it go far enough? Meeting the definition of AI above would imply that the AI has to go beyond this and create new solutions to trying situations or solutions to entirely new situations. But an AI trains on data and finds patterns, memorizing the data only allowing it to return the data to the user in a new format, within the knowledge given in the training data. This limitation of AI keeps it from being a true intelligence and more of a mimic of intelligence, just returning the intelligence of those who created the information within the data set.
We see this same understanding in industry now to discover when and where to use AI tools that also begs an important question:
If AI is only a data set reflection, if it is only a trend within data, how far can we take it? This is a dangerous question, we would all like to predict the future but how can we be certain of the answer? In the past there have been scientists sure that something was impossible, see supersonic flight, only to find that the trend did not extend past the data set they currently had.
There’s a nuance and a need for discovery of what the limits are of AI – Many adopters can claim that AI is near omniscient and perfect, only because it produces authoritative sounding answers. Others may rightfully claim that the mathematically honest answer is AIs can only reliably function within their training data set. Practical appliers, though, know that just outside the training data set, there’s a regime where the model will still produce reasonably good results, and we can use those results to accelerate our progress to the new correct answers for a future training set.

Within the training data set, all of these answers are reasonably accurate and you can dial up the order to get a better fit. What is wildly different is what happens outside this known set of data. The 3rd order fit will continue to rise to infinity, while the 9th order curve will slam to zero (or into a median on the highway at 80mph). Which AI do you trust to guess the future? This is the risk facing the trust of AI outside of its training data.
Large Language Models (LLMs) are the peak of AI today, at times interacting with a LLM can feel like interacting with another human. To really break down what is happening though is to look at the extreme, turning the dial up on the accuracy of the LLM to 100% and the conversational responses become direct copies of the data it learned from. This proves that the LLM is a memorization tool, when it is further from the extreme it is just transforming the data, not “dealing with new or trying situations”.
What is Simulated Intelligence?
LLMs are a great tool but like with any tool understanding how it works and its limitations allows us to optimally use the tool. The LLM is really a simulation of intelligence, parroting results in a transformed manner that is more conversational. The original knowledge is still the knowledge that comes through, and that knowledge is from the intelligence of the creators, the authors, the artists, the scientists.
With the emergence of new technology there can be a lot of misinformation and with that a lot of lost opportunities. With a better knowledge of the technology and what it does, there can be better business opportunities pairing to AI. Understanding the power of the tool and what its limitations are allows a business to be built around it without promising results that are unattainable. Understanding allows for the development of more powerful products and the weeding out of ideas that clearly are not feasible.
WHAT IS THE GOAL OF AN AI COMPANY?
A lot of companies have developed very powerful AI’s, but they have yet to find a profitable business model. What is more successful in today’s market is using these AIs as a tool to improve or differentiate your product from the competition. Companies like Microsoft use AI in Bing and Word to improve the experience. Using a generative AI to produce images is a cool idea, but it’s a powerful addition to a photo editor. The examples can be numerous and there are tons of potential for AI to add to current product lines and allow companies to use traditional business models.
How a company leverages AI to build a product or enhance a product is essential to success. Understanding the capabilities and deploying them to build and enhance products beyond the base capability is what gives utility. With AI, think about the application; increasing the efficiency of producing products, improving the workflow for clients, and adding novel features for clients. Can you make the next success story like Bing and chatGPT?
What is the role of AI in manufacturing?
The question of how does AI fit and enhance manufacture is an important one. How does AI enhance our manufacturing capability? It may not be as straightforward as another app, as manufacturer’s products often have no computers as a part of the product. Is AI’s benefit within design? Can it control hardware better and more efficiently – and be implemented less expensively than modern control systems like PID? Finally, how to verify and validate AI in a hardware context is necessary – as many of these systems are safety critical or have an impact to life. The cost of that verification and validation of an (often) black box AI in money and time may be prohibitive and is worth investigating before starting down that path. We’ll be exploring these questions and more in the next AI blog.